
AI Pricing: strategies for growth and profitability
AI is booming, revolutionizing industries and reshaping the way businesses operate. As organizations increasingly adopt artificial intelligence technologies, they face unique pricing challenges that can significantly impact their success.
This article examines AI pricing challenges and offers practical insights from industry leaders. You will learn effective strategies to balance profitability with customer satisfaction, along with actionable takeaways from real-world examples.
Let’s delve into it!
The true challenges of AI product pricing
AI product pricing is fundamentally different from traditional software pricing due to several factors, if you recognize at least one of them as your challenge, you’re on the right article to find your answers:
- Rapid usage growth and automation demands: AI products often experience exponential growth in usage, particularly as automation increases. This can lead to unpredictable cost structures and resource allocation challenges.
- High costs of LLMs and GPUs: The underlying infrastructure for AI, including large language models (LLMs) and GPUs, comes with significant costs. These expenses must be factored into pricing models to ensure profitability in time.
- Customer need for predictable spending: While AI usage can be variable, customers still desire predictable costs for budgeting purposes. This creates tension between usage-based pricing and fixed-cost models.
- Upfront costs to serve demand: AI companies typically face substantial upfront investments to meet potential demand, which can strain cash flow if not properly managed through pricing strategies.
Why traditional pricing models fall short for AI?
Traditional software pricing model often proves inadequate for AI products. Seat-based pricing, a staple in enterprise software, doesn't align well with AI usage patterns.
AI products frequently see most usage coming from a small number of power users or automated processes, rather than being evenly distributed across all seats.
This misalignment can lead to underpricing and margin erosion. On the other hand, while flat-rate plans offer simplicity and predictability, they struggle to account for the highly variable consumption rates typical in AI applications. Companies often find themselves either leaving money on the table with heavy users or pricing out potential customers with lower usage needs.
What is an effective AI pricing strategies?
We identify 4 patterns of successful pricing model in the market for AI companies. In this article, we will examine how these models operate and why they effectively address the complexities of AI pricing.
Usage-based pricing:
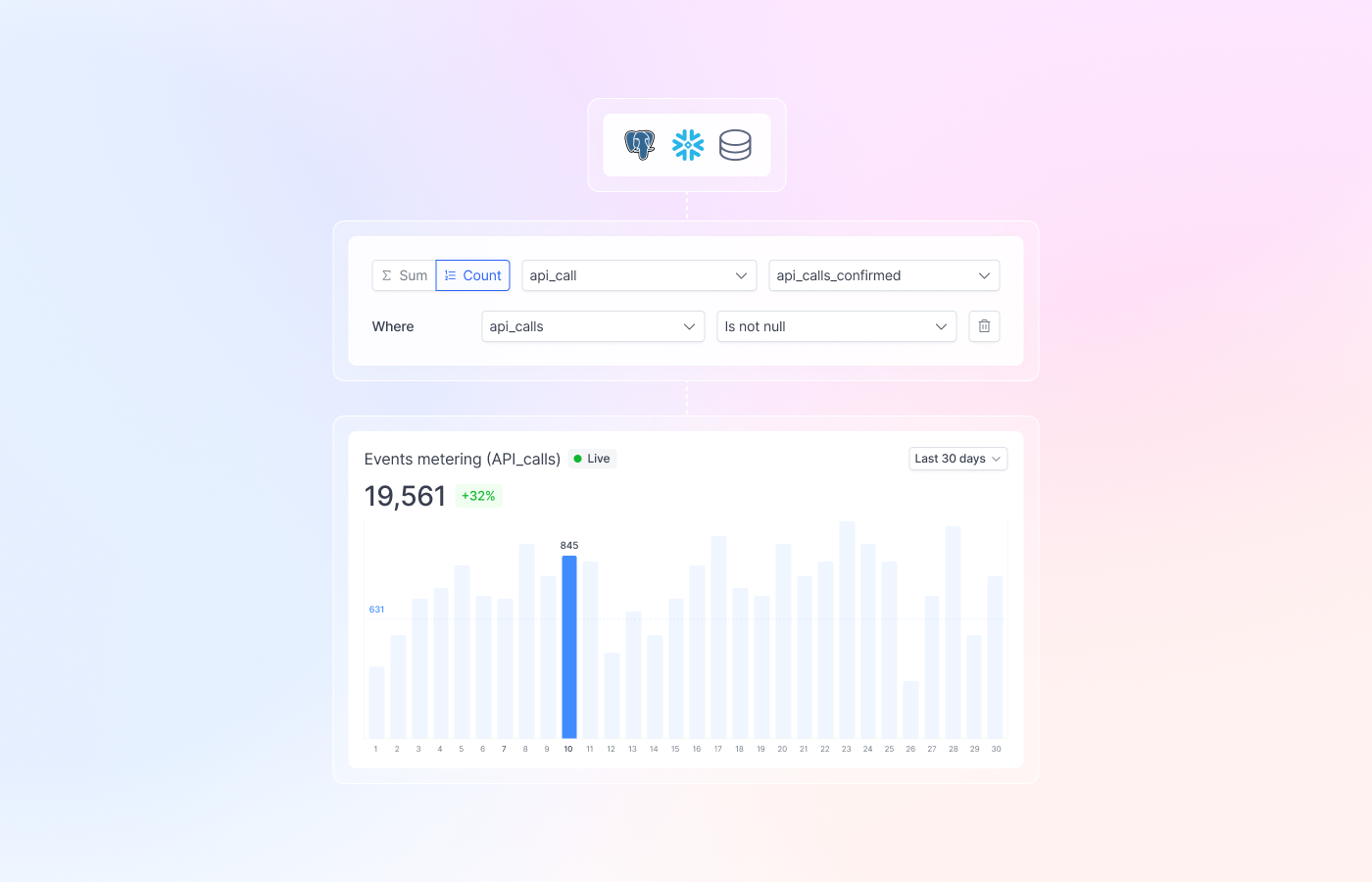
Example: OpenAI (ChatGPT).
Strategy: OpenAI initially used a post-consumption billing model based on token usage. In February 2024, they shifted to a prepaid model where users purchase credits in advance.
Why it works: This approach aligns costs directly with usage, allowing OpenAI to manage its infrastructure costs effectively while giving users control over their spending. The prepaid model also helps with cash flow management.
Cost-plus pricing:
Example: anthropic (Claude AI).
Strategy: anthropic uses a cost-plus model for its Claude AI, charging based on the number of tokens processed with different rates for input and output tokens.
Why it works: This transparent approach allows anthropic to maintain consistent margin while giving customers a clear understanding of their costs. It also enables anthropic to adjust prices as underlying costs change.
Bring your own API key (byok):
Example: Langchain.
Strategy: Langchain allows users to bring their own API keys from providers like OpenAI or anthropic, charging only for the Langchain platform usage.
Why it works: This model appeals to developers and companies who want more control over their AI costs and may already have relationships with foundational model providers. It also allows Langchain to focus on its value-add without managing the underlying API costs.
Success-based pricing:
Example: Forethought AI.
Strategy: forethought, which provides AI for customer support, charges based on the number of successfully resolved tickets rather than raw usage.
Why it works: This aligns the cost of the AI service directly with the value it provides to the customer, making it easier for customers to justify the expense and potentially leading to higher customer satisfaction.
Hybrid models and credit systems
Many companies are finding success with hybrid approaches because these models combine the strengths of different pricing strategies, allowing for greater flexibility and adaptability.
Hybrid models can reduce the risks associated with relying on a single pricing structure, enabling companies to respond more effectively to market changes and customer feedback. This adaptability fosters customer satisfaction and loyalty, ultimately driving long-term success.
Here are a few examples:
Combining seat-based plans with usage-based AI components:

Example: Intercom.
Strategy: intercom integrates AI capabilities into its existing customer communication platform. They charge a base subscription fee for the platform and additional fees for ai-powered resolutions.
Why it works: This allows intercom to introduce AI features without overhauling their entire pricing structure, while still capturing the additional value provided by AI.
Hybrid pricing strategy with AI add-ons
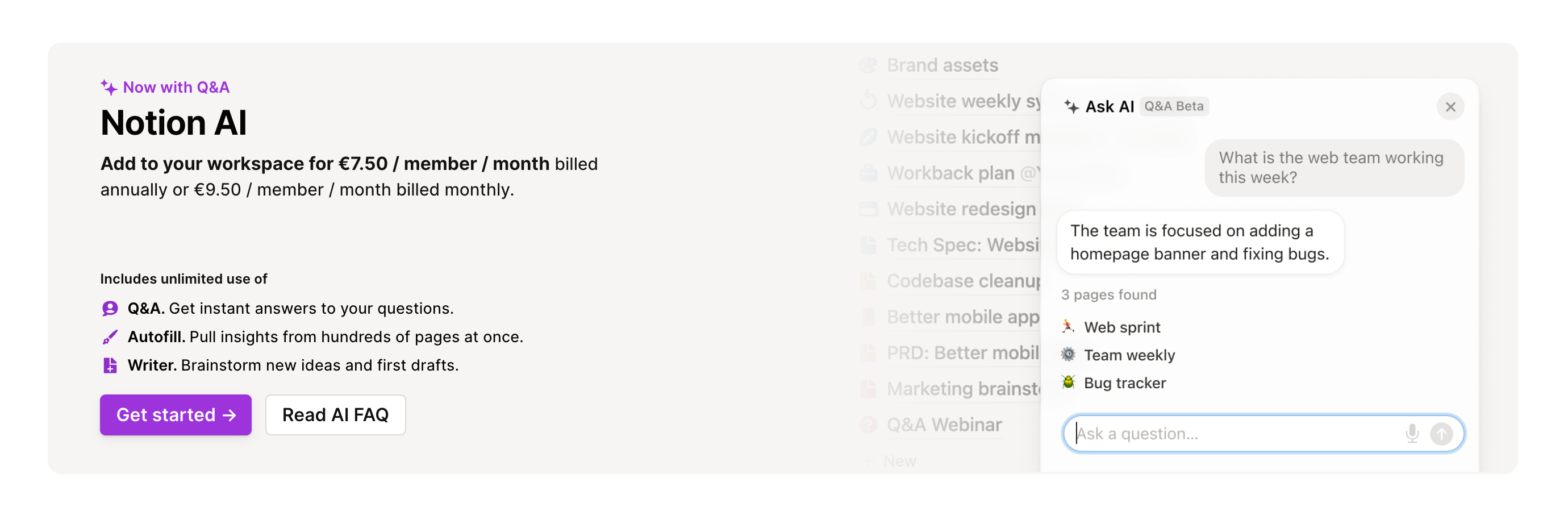
Example: Notion AI.
Strategy: Notion offers a hybrid pricing model that includes a base subscription with optional add-ons, such as AI features, for a small additional fee.
Why It Works: The low-cost AI add-on makes it accessible, encouraging users to try it without a significant financial commitment.
Implementing credit systems and usage limits:

Example: jasper AI.
Strategy: Jasper offers tiered plans with monthly word credits. Users can purchase additional credits if needed.
Why it works: This model provides predictability for users with consistent needs while allowing for flexibility for those with variable usage. It also encourages users to upgrade to higher tiers as their usage increases.
Prepaid billing strategies:
Example: copy AI.
Strategy: The company uses a prepaid credit system where users purchase credits in advance and can set up auto-refills.
Why it works: This approach helps copy AI manage cash flow and reduces transaction costs. For users, it provides better spending control and can psychologically feel less expensive than a recurring subscription.
How to build an AI pricing strategy?
Building an AI pricing strategy requires a thoughtful approach that considers various factors unique to AI products.
Understanding AI costs
Start by thoroughly understanding your costs. This includes infrastructure costs like GPU usage, API calls, and data storage, as well as development and maintenance costs.
Next, analyze your target market and customer segments. Understand their willingness to pay, usage patterns, and value derived from your AI product. This information will help you determine the most appropriate pricing model and price points.
Develop the right pricing model for your business
Consider the various pricing models discussed earlier, such as usage-based pricing, cost-plus pricing, or hybrid models. Evaluate which model aligns best with your cost structure, customer needs, and business goals. Remember, the right model can vary depending on your specific AI application and target market.
Develop a pricing structure that balances profitability with customer value. This might involve for example credit systems, or success-based pricing. For instance, if you notice certain features driving higher costs, you might price those separately or include them only in higher tiers.
Choose the right recurring billing system for your strategy
Implement tools for monitoring and managing your pricing strategy. Hyperline can play a crucial role here. Its real-time data tracking allows you to continuously monitor the profitability of your pricing strategy and make data-driven adjustments as needed.

Plan for scalability and future adjustments. The AI business is rapidly evolving, and your pricing strategy should be flexible enough to adapt. Regular reviews of your pricing strategy, informed by data from tools like Hyperline, can help ensure your pricing remains competitive and profitable as your AI product evolves.
A/B test and Iterate quickly and easily your pricing plan
Test your pricing strategy before full implementation. This could involve A/B testing different pricing models with a subset of customers or running simulations based on historical usage data.
Finally, be prepared to iterate. AI pricing is still a relatively new field, and what works today might need adjustment tomorrow. Continuously gather feedback from customers and analyze performance data to refine your pricing strategy over time.
One of the key advantages of using Hyperline is the ease with which companies can change pricing and test new pricing strategies. Hyperline’s intuitive interface allows businesses to modify pricing structures quickly, whether implementing discounts, introducing new tiers, or adjusting subscription fees. This flexibility enables companies to respond swiftly to market feedback and customer needs.

To conclude
Throughout this process, a tool like Hyperline serves as an invaluable partner. For AI businesses, managing billing and collecting data from clients can be challenging without such a tool.
The highlights of using Hyperline dashboards include the ability to track income, churn rates, and new payers effectively. By streamlining the billing process and gathering essential data, Hyperline enables businesses to identify trends, optimize pricing strategies, and enhance customer management. This level of visibility is crucial for understanding customer behavior and making data-driven decisions.
Q&A About AI pricing
Q: What successful AI pricing strategy are made of?
Successful AI pricing strategies must navigate the tension between covering high infrastructure costs, providing predictable spending for customers, and remaining competitive in a fast-moving market.
Q: What future directions can we expect in AI pricing models?
As AI capabilities expand, we can expect more nuanced pricing models that tie costs to specific AI functions or outcomes. Additionally, there may be increased differentiation between pricing for human-guided versus fully automated AI usage.
The next gen of monetization platform
Set it up once and never think about billing again.